Using Machine Learning to identify key biomarkers
Biomarker discovery is essential for molecular diagnostics. Machine Learning (ML) improves biomarker identification and validation for diagnostic applications.
Challenge
The client needed a robust ML framework to analyze omics data from 700–800 patient samples, with 7,000–8,000 gene expression features and 2,000 SNP features.
Approach
- Performed multi-stage, nested cross-validation.
- Used feature engineering based on interaction networks.
- Evaluated and delivered the top three ML models for validation.
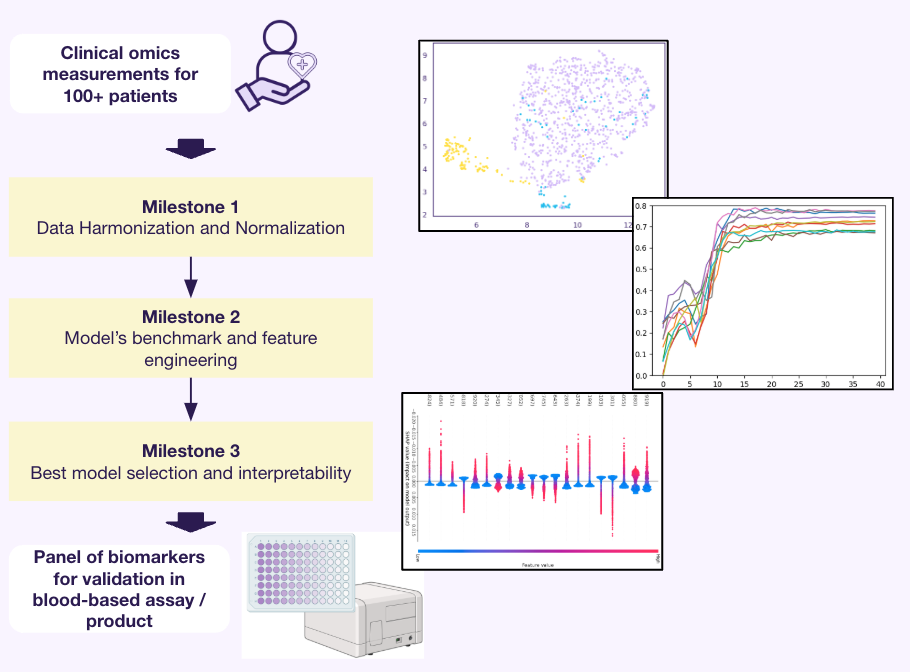
Results
- Established a panel of biomarkers validated for blood-based assays.
- Delivered ML models with superior predictive performance.
Artificial Intelligence-based biomarker discovery is boosting advances in diagnostics, providing accurate and reliable disease detection.