How did a leading U.S. pharmaceutical company improve AI model management for hypothesis generation?
AI-based knowledge graphs are transforming hypothesis generation in pharmaceutical research, but effectively managing AI workflows and datasets remains a challenge. For a leading U.S. pharmaceutical company, it implemented AI Lab Loop, an AI platform for managing workflows and datasets, to improve the performance of its knowledge graph model. This case study explores how AI Lab Loop simplified training, benchmark testing and deployment of AI models, leading to more efficient and automated hypothesis generation.
Challenge
- Improving the performance of a knowledge graph model used for hypothesis generation.
- Simplifying and automating the process of training and benchmarking AI models.
- Providing an intuitive interface for uploading and managing training datasets.
- Enabling continuous model training and easy comparison between AI models.
- Automating the transition of selected AI models from development to production.
Our Approach
- Implemented AI Lab Loop, a web-based AI workflow and dataset management platform, to streamline model training
- Enabled automated AI model benchmarking, allowing for optimal model selection
- Developed a user-friendly interface for seamless dataset uploads and management
- Integrated continuous training capabilities to improve model performance over time
- Implemented automated model promotion, ensuring efficient deployment of selected AI models to production
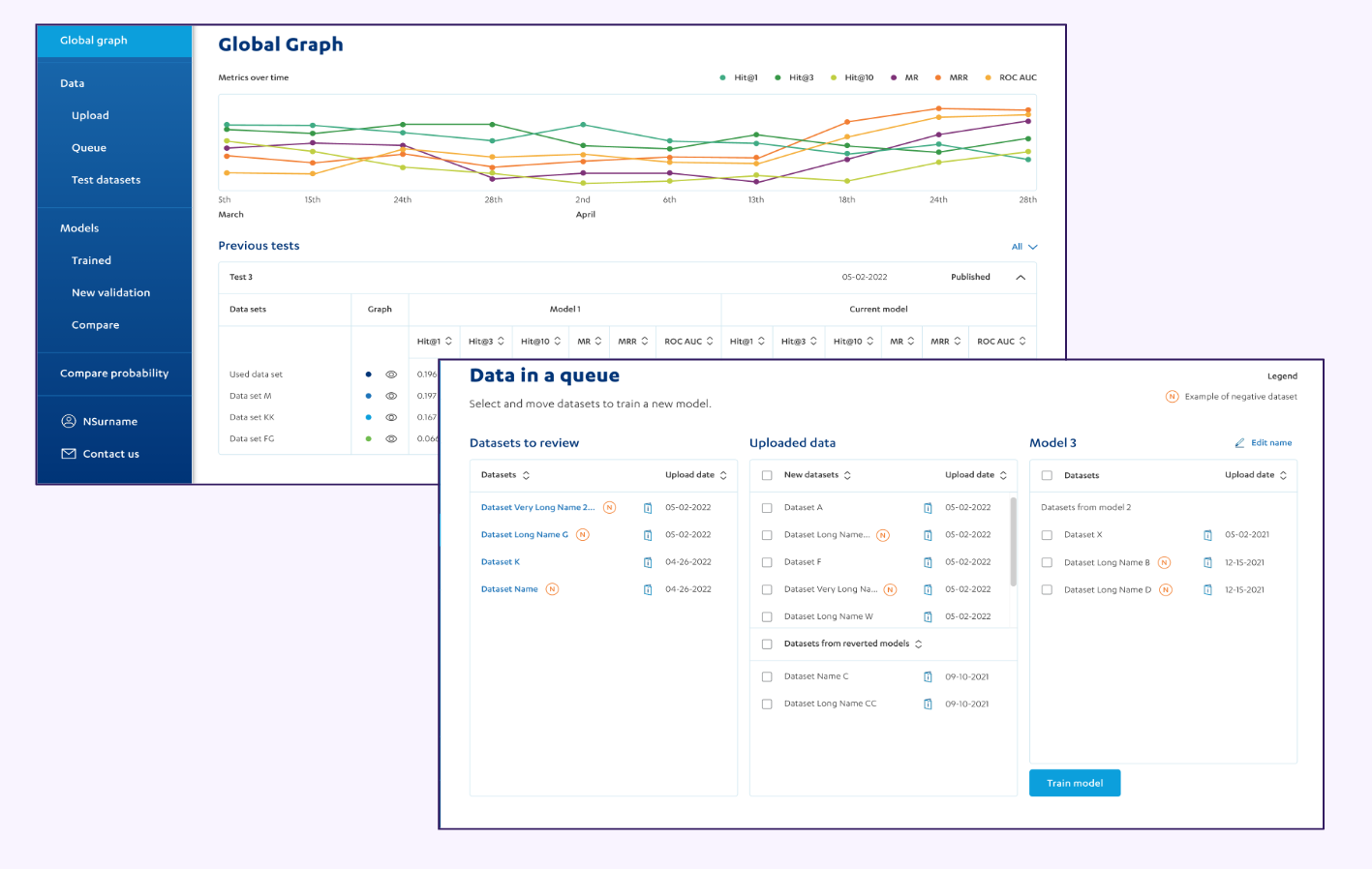
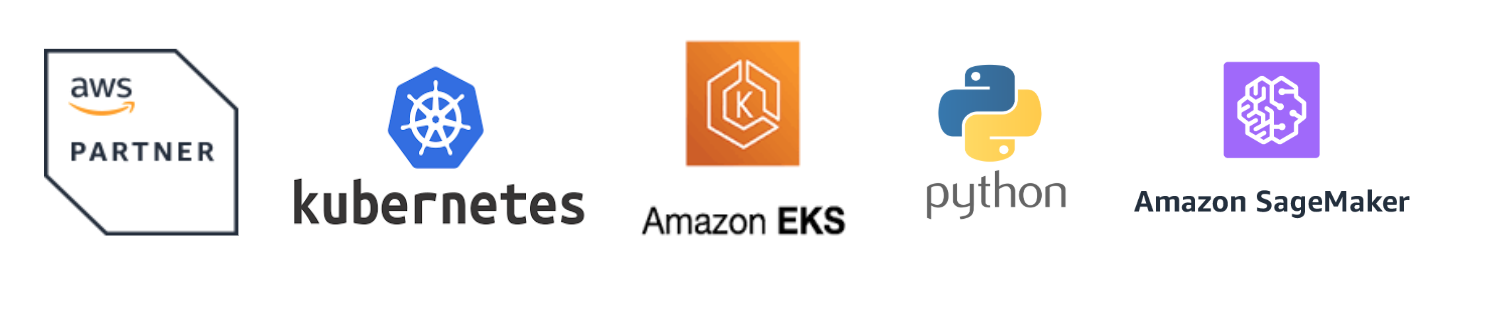
Technology stack
Results
- Data-Driven Experimentation: AI model results now directly inform experiment design
- Optimized Model Selection: AI Lab Loop simplifies benchmarking, ensuring the best model is chosen efficiently
- Automated Deployment: Selected AI models seamlessly transition from development to production
- User-Friendly Dataset Management: Researchers can easily upload and manage training datasets
- Continuous Improvement: Ongoing model training and comparisons enhance AI-driven hypothesis generation
Using AI Lab Loop, the pharmaceutical company has successfully optimized its knowledge graph model training process. The platform automates model selection, implementation and continuous learning, enabling more efficient hypothesis generation. With a user-friendly interface and automated workflows, researchers can now focus on scientific discovery rather than AI model management, driving innovation in pharmaceutical research.