How did Ardigen help a global pharmaceutical company identify the most accurate in vitro cancer models using Multi-Omics and AI?
The success of cancer research depends on using appropriate in vitro models – ones that truly reflect the biological complexity of patients’ tumors. But selecting the most representative models from a sea of cancer cell lines is no small feat. In this case study, we show how Ardigen supported a major pharmaceutical company in improving its model selection process by matching cell lines to patient samples using state-of-the-art machine learning and omics data harmonization.
Challenge
The pharma client’s Target Sciences and Functional Genomics Group needed to pinpoint which in vitro models best mirrored real patient tumor biology. They faced:
- Difficulty comparing gene expression between cancer cell lines (CCLE) and patient tumors (TCGA).
- Data inconsistency and batch effects across datasets from different sources.
- A lack of direct mapping tools for evaluating model-to-patient similarity at the molecular level.
Approach
Ardigen developed and executed a custom analytical pipeline to address these challenges, combining advanced machine learning and biological insight:
- Harmonization of CCLE and TCGA gene expression profiles, resolving inconsistencies between datasets.
- Algorithmic mapping of patient profiles to cell lines, enabling accurate, one-to-one biological comparison.
- Use of Variational Autoencoders (VAE) for precise sample alignment between in vitro and in vivo data.
- Tailored batch correction strategies to ensure reliable, consistent analysis across datasets.
- Adversarial training techniques to enhance the model’s precision and robustness in differentiating subtle biological patterns.
Schematic diagram of a solution
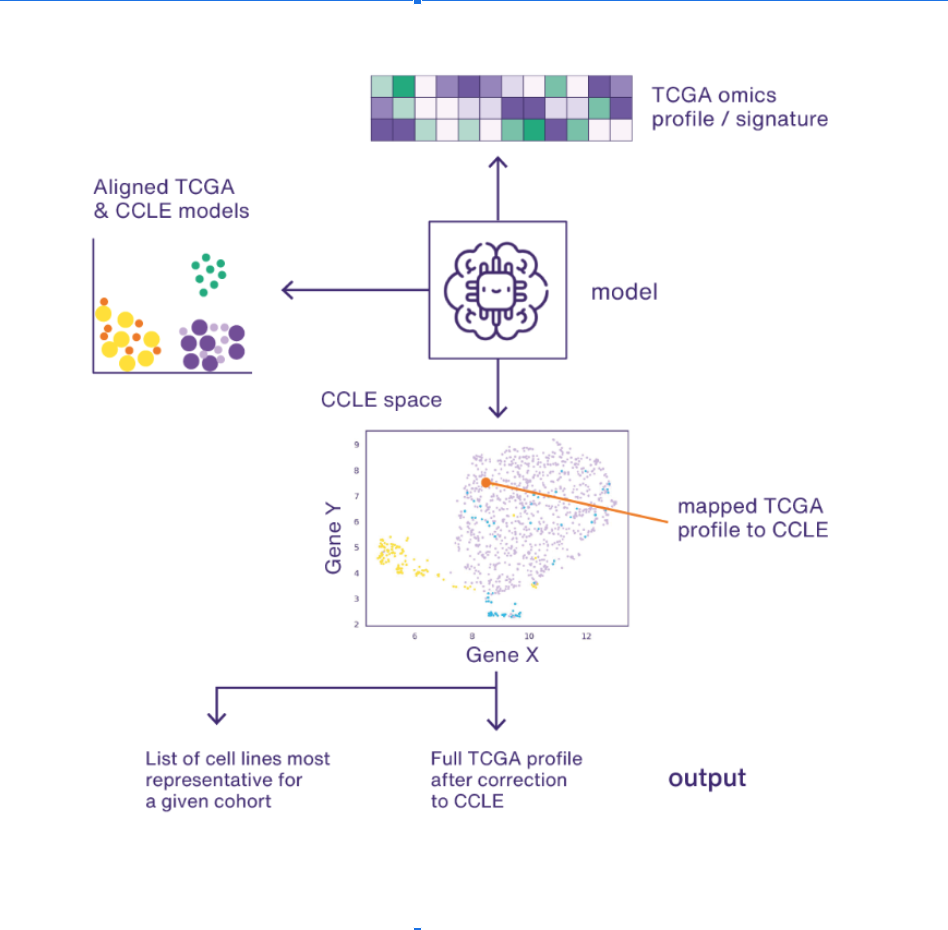
Results
The project delivered multiple high-impact outcomes:
- Reliable harmonization of TCGA and CCLE profiles for accurate model selection.
- Patient sample mapping that supported biologically grounded comparisons across datasets.
- Development of an optimized workflow for selecting in vitro models specific to cancer types and subtypes.
- Increased accuracy in model matching via VAE alignment and adversarial refinement.
Through expertise and technological innovation , the client has gained a robust framework for selecting in vitro models that accurately reflect the biology of a patient’s tumor. This capability has become an important asset in their R&D process, accelerating cancer research and improving the accuracy of experimental results.