Drug development is increasingly dependent on data. From clinical trials and patient monitoring to personalized medicine, the volume and variety of information generated today is unprecedented. Yet, managing all this data effectively remains a significant hurdle. Many organizations face challenges integrating disparate datasets, analyzing information in real-time, and ensuring data quality and compliance. A clinical data lakehouse offers a fresh approach to solving these problems by combining the strengths of two existing data architectures: data lakes and data warehouses. This hybrid model helps organizations handle complex drug development data while unlocking new opportunities for research and patient care.
Understanding the clinical data lakehouse system
A clinical data lakehouse is a data management system that merges the scalability of data lakes with the structure and governance of data warehouses. Data lakes excel at storing vast amounts of raw, unstructured data, while data warehouses are optimized for structured, organized data that is ready for analysis. A lakehouse combines these capabilities, allowing drug development organizations to store all their data in one place while still making it accessible for advanced analytics, machine learning, and real-time decision-making.
For drug development and life sciences companies, this means they can work with data from various sources—such as electronic health records, genomic studies, imaging systems, and wearable devices—without compromising on quality or accessibility. Unlike traditional systems that struggle to integrate unstructured data, a clinical data lakehouse provides a unified platform for storing, managing, and analyzing both structured and unstructured information.
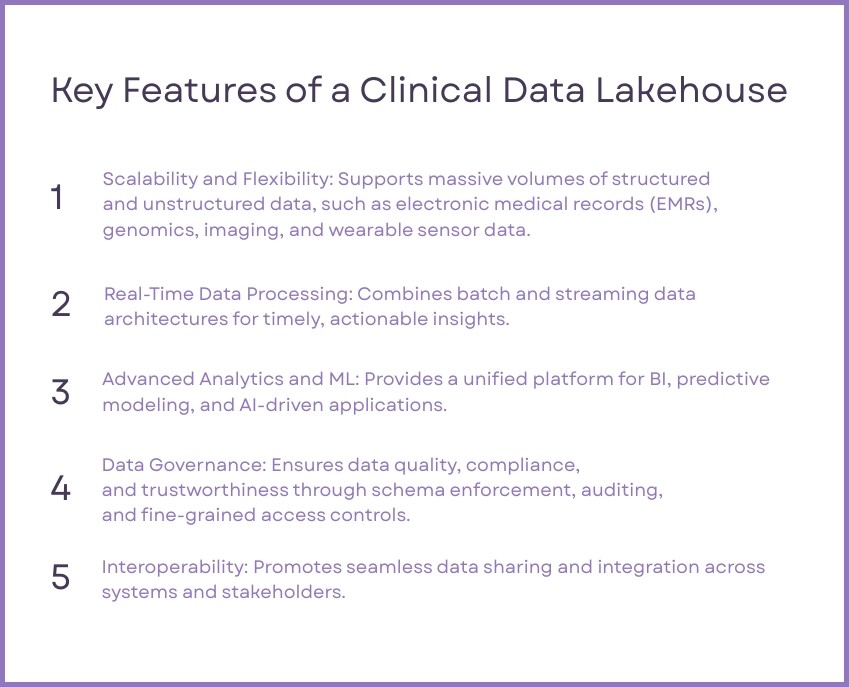
Key Features of a Clinical Data Lakehouse
Why clinical data lakehouses are imperative now
The need for clinical data lakehouses has never been greater, driven by the growing complexity and scale of healthcare data. Modern healthcare systems generate immense volumes of data from sources such as clinical trials, wearable devices, and electronic medical records [1]. That data isn’t just vast in quantity—it’s also diverse, encompassing structured lab results, unstructured imaging, and real-world evidence like social determinants of health. A clinical data lakehouse consolidates these disparate data types into a single, accessible platform, enabling organizations to manage both real-time and historical data effectively.
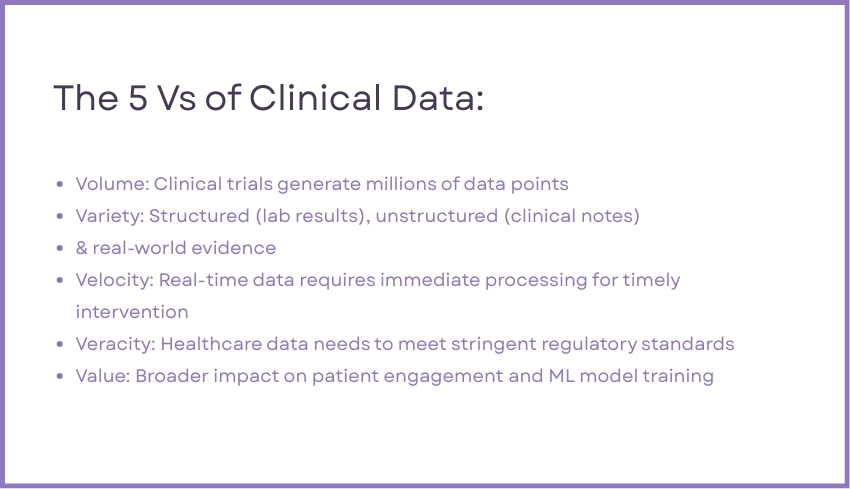
The 5 Vs of Clinical Data
Traditional architectures, which rely on separate systems for raw data storage and analytics, often struggle with inefficiencies like data duplication, latency, and siloed information. These limitations delay insights and increase operational costs. By unifying raw and processed data on a single platform, clinical data lakehouses eliminate redundancies and support real-time analytics. This approach empowers drug development providers to make faster, more informed decisions, whether it’s predicting ICU demand or tailoring interventions for individual patients.
Beyond operational efficiency, clinical data lakehouses unlock transformative applications in areas such as drug discovery and population health management. They enable large-scale genomic analyses, support proactive care through aggregated data insights, and foster innovation by integrating AI and machine learning capabilities. With robust data governance and compliance measures built in, lakehouses are not just a technological upgrade—they’re a crucial foundation for successfull drug development.
The benefits of a clinical data lakehouse
A clinical data lakehouse streamlines drug development data management by unifying all types of data—structured and unstructured—into a single repository. Tools like Delta Lake optimize performance, simplify ingestion, and provide connectors for domain-specific data. This centralization fosters collaboration among diverse teams, enabling data scientists and clinical researchers to work together seamlessly. The platform’s support for common programming languages like SQL, Python, and R enhances accessibility, encouraging innovation across disciplines.
By combining historical and new data, lakehouses enable real-time insights essential for interventional care and personalized medicine. Advanced governance features, such as schema enforcement and auditing, ensure data integrity and regulatory compliance, while AI model tracking bolsters trust and reproducibility. Moreover, cloud-native scalability allows organizations to handle growing data demands cost-effectively, eliminating the limitations of traditional on-premises systems.