Using AI to revamp peptide bond design for faster and more effective drug discovery
High-affinity peptide binding design has traditionally relied on labor-intensive screening techniques, such as phage display, which can be costly and time-consuming. This case study explores how an AI-supported de novo design pipeline enabled a biotech client to efficiently generate, evaluate and prioritize peptide candidates, significantly speeding up the discovery process while optimizing laboratory resources.
Challenge
A client approached with the challenge of developing a pipeline capable of designing peptides that specifically bind to a target of interest. The core need was to move beyond traditional screening methods and leverage computational power to generate and prioritize potential peptide binders efficiently. The client aimed to avoid the time and resource intensive process of screening large random libraries using methods like phage display.
Approach
The presented case study outlines the development and implementation of an AI-based de novo design pipeline for target-specific, high-affinity peptide binders (8-15 amino acids). This pipeline features a modular setup of functional components designed to streamline the discovery process. The approach begins with an AI-based generator that designs initial binder candidates based on the target structure. Subsequently, a cascade of filtering steps is applied to these candidates. This downstream filtering and ranking process incorporates predictions for interaction quality, solubility, and toxicity, ensuring that only the most promising peptides advance.
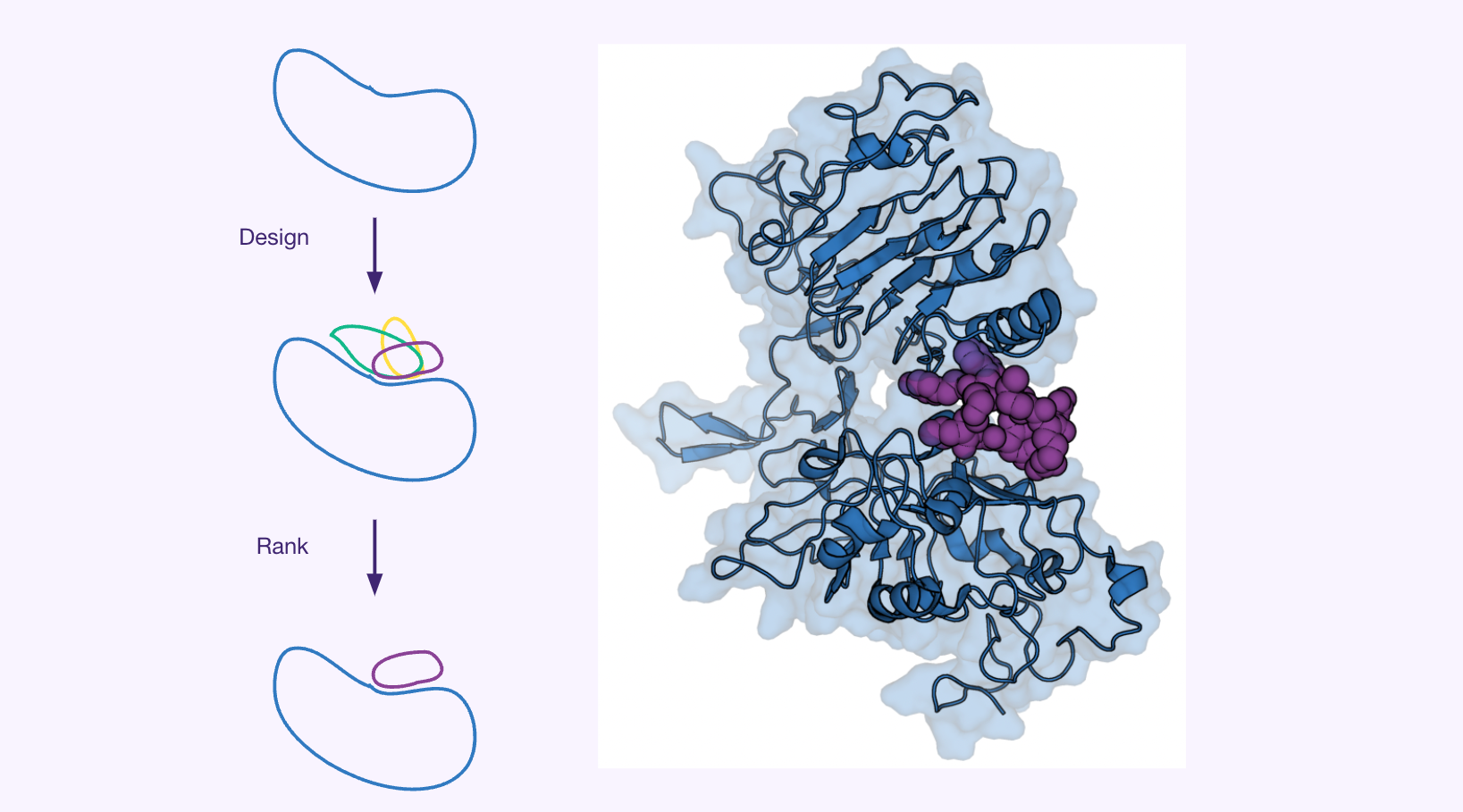
Results
- More candidates scanned: explored a larger sequence space than traditional methods.
- Enlarged sequence space: AI design accessed previously unreachable regions.
- Optimized lab resources: focused wet-lab work on promising, pre-selected candidates.
- Accelerated discovery: easy scaling of in silico experiments enabled rapid evaluation.
By implementing a de novo design pipeline based on artificial intelligence, the client has successfully overcome the limitations of traditional peptide screening methods. The approach expanded the sequence space under study, optimized efforts in wet labs, and enabled rapid evaluation of potential binding agents on a large scale. In summary, this case study highlights the successful development and implementation of an AI-based platform for de novo peptide binding design. By integrating AI-based generation with intelligent filtering, the client was able to bypass traditional high-throughput screening methods, leading to more efficient, resource-optimized and accelerated discovery of potential therapeutic peptide candidates. This demonstrates how AI-based computational design can revolutionize peptide discovery, making the process faster, more efficient and highly scalable.