Applying artificial intelligence to optimize therapy with antibodies with increased binding affinity
Traditional antibody discovery methods, such as phage display, often yield bindings with suboptimal affinity, requiring intensive laboratory work for further optimization. This case study examined how an AI-based lead optimization strategy enabled a biotech client to more effectively refine antibody candidates. Using statistical analysis and large language models (LLMs), the client was able to identify key binding regions, generate improved sequences and increase binding affinity – accelerating the development of high-quality therapeutic antibodies.
Challenge
A client, utilizing traditional methods like phage display, had identified approximately 100 antibody binders for a target of interest. However, the measured binding strength of these binders was insufficient for their therapeutic goals. They sought a solution to leverage AI to generate a refined, smaller pool of binders with significantly improved binding affinity.
Approach
The approach implemented was an iterative AI-driven lead optimization strategy. The process began with a statistical analysis of the existing 100 binders to identify key sequence regions crucial for target binding and interface formation. This analysis provided valuable insights for targeted modifications. Subsequently, multiple Large Language Models (LLMs), trained on extensive protein datasets and specialized antibody data, were employed to design improved antibody sequences. This computational approach enabled the exploration of a vastly larger sequence space than feasible through traditional wet-lab methods. The plan was to iteratively test pools of these AI-generated binders, assessing the impact of proposed changes on binding affinity and refining the design process accordingly.
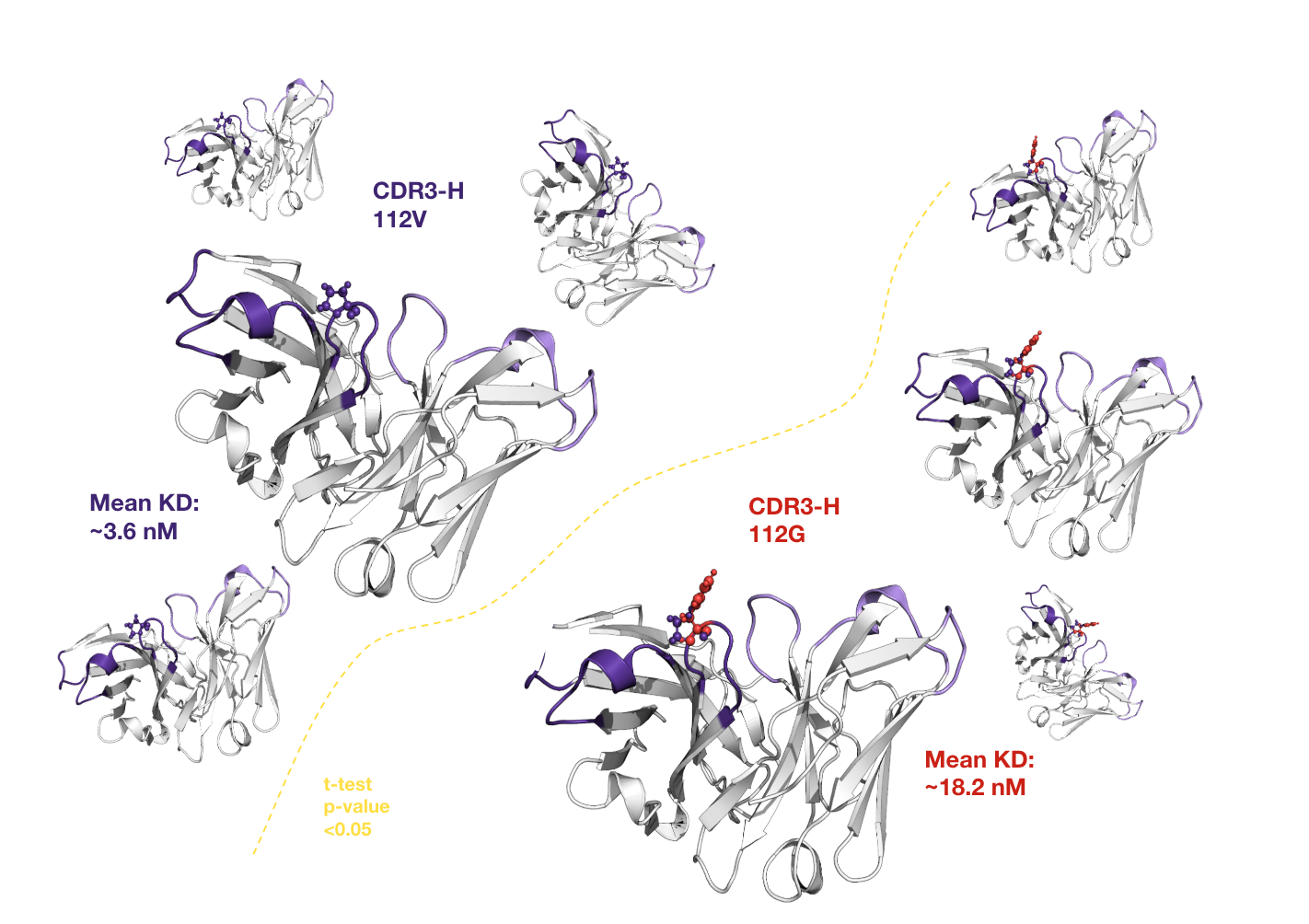
Results
- Expanded candidate pool: AI explored a larger sequence space.
- Resource efficiency: reduced lab work, accelerated development.
- Enhanced affinity: iterative, data-driven design improved binding.
By implementing an AI-led lead optimization strategy, the client successfully refined the initial pool of binding antibodies, significantly increasing binding affinity while reducing reliance on resource-intensive laboratory methods. The approach used statistical analysis and large language models (LLMs) to identify key binding regions and generate improved sequences, expanding the candidate space studied beyond traditional methods. In essence, this case study demonstrates the effective use of artificial intelligence, particularly statistical analysis and LLM, to overcome limitations in traditional antibody discovery. By integrating iterative data-driven design, the client has achieved a more efficient, scalable and targeted approach to developing high-affinity therapeutic antibodies.