Abstract
Understanding microbial proteins is essential to reveal the clinical potential of the microbiome as a whole. The application of novel high-throughput sequencing technologies allows for the fast and inexpensive acquisition of all potential protein sequences from a microbial community. However, many of these sequences do not resemble those of previously characterized proteins. Therefore, predicting their function through conventional alignment-based approaches is challenging. Recent research has shown that deep learning – a collection of prominent Artificial Intelligence methods – may be the missing piece in solving this problem. This is the first part of the series, which aims to advocate for the use of deep learning in metagenomics and will be soon followed by part two – a brief review of recent advances in deep learning-based protein function prediction.Introduction
The human gut is colonized by millions of commensal microorganisms, which makes it one of the most diverse ecosystems known. The gut microbiome is called the new “hidden” human organ due to the vast genetic potential of these organisms as well as their metabolic and biosynthetic capabilities. A growing body of evidence links the gut’s dysbiosis with diseases like diabetes, inflammatory bowel disease, cancer, or even autism, showing the microbiome’s profound impact on human health. However, we still lack a detailed understanding of these communities and mechanistic explanations of their role in developing diseases [1] [2]. Taxonomic profiling through 16S rRNA sequencing is possibly the most prevalent method for characterizing the human gut microbiota. By providing gut community microorganism composition, these studies have revealed unexpected correlations between bacteria and the host’s health. However, unrelated bacterial families can maintain analogous metabolic activities, making it difficult to infer functional mechanisms underneath phylogenetic associations. For this reason, the field is currently shifting its focus to the functional profiling of human microflora [1, 2]. Nevertheless, functional profiling is more challenging, as it requires the use of whole-genome sequencing (WGS) to sequence hundreds of thousands of genes from all microorganisms in a given sample. Genes encode proteins which each implement a biological function. The issue is that we are unable to deduce the function of more than 50% of all microbial proteins’ sequences. Despite remarkable progress in the last few decades, developing precise methods for functional prediction is still a major challenge in bioinformatics (see CAFA [3] and CASP [4] initiatives). The volume of metagenomic data is making the problem even harder. A powerful in silico method for predicting protein functions will have enormous benefits, not only for metagenomics.Predicting protein functions
The function of a protein is a direct derivative of its amino acid sequence. Currently, the general procedure for identifying a protein’s function is to compare a sequence of a novel protein to all experimentally examined sequences stored in multiple databases. BLAST [5] is the most popular tool for performing elemental sequence alignments. More advanced tools (e.g., PSI-BLAST [6], HMMER [7], HH-suite [8]) leverage multiple alignments to build models that find sequence patterns, such as profiles or motifs, and represent them as Position Weight Matrices (PWM) or Hidden Markov Models (HMM). These profiles can then be utilized to search databases iteratively to detect distant homologies, enabling the discovery of protein clusters or families that are evolutionarily connected. The alignment scores can help indicate the degree of sequence similarity between the novel sequence and existing database sequences. The above described approaches are both popular and powerful for protein function annotation directly from a protein sequence. However, these approaches are still limited in classifying sequences of proteins with similar function or structure, but distant in the sequence space. To illustrate, Cas9 and Cpf1 are both Class II CRISPR effector proteins with very similar functions, but they have very different domain architecture and share only ~15% amino acid identity. Existing approaches fail to reveal their analogous functions just by comparing their amino acid sequences [9]. Furthermore, nearly every novel metagenomic dataset contains new proteins with unique sequences. Despite the massive growth of databases in recent years, rich microbial diversity and rapid evolution make it impossible to catalog all proteins existing in nature. Moreover, current methods may not be able to handle such a volume of data. Therefore, we should shift our approach from comparing proteins to enormous databases to developing tools that can learn from these databases and draw functional conclusions.Deep learning
Deep learning is a proven technique to solve intricate problems and has been shown to work exceptionally well for tasks such as speech recognition, natural language processing (NLP), and image classification. Recently, it has been successfully utilized to analyze biological sequences like genomes or proteomes [10]. The most well-known example is DeepMind’s AlphaFold model [11, 12], which dominated the last protein structure prediction challenge – CASP13 [13]. However, there are many other related domains where deep learning is quietly becoming a standard, such as the prediction of transcription factor binding [14], de novo drug design [14], and base calling in nanopore sequencing [15]. The main reason for the tremendous success of deep neural networks in these fields is their ability to process massive amounts of data, even unlabeled, and learning meaningful patterns within them. Deep learning can handle, and even leverage, the exponential growth of data available in biological databases, which is a challenge in traditional methods. In (meta)proteomics, the ability of deep neural networks to learn from unlabeled data is particularly valuable, as the gap between the number of unlabeled and labeled proteins is widening every year [Figure 1].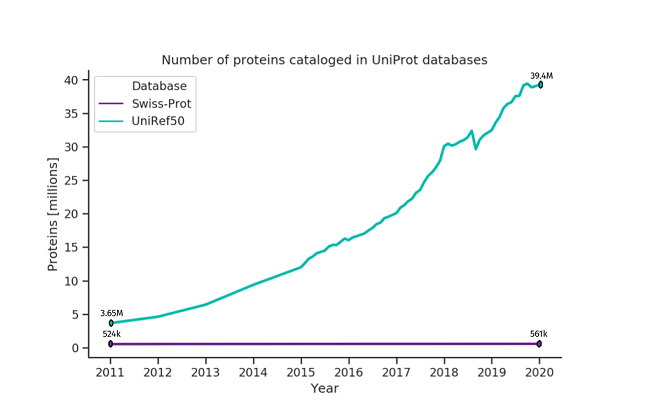
Summary
- Understanding of microbial proteins is crucial for unlocking the microbiome’s clinical potential.
- Developing a precise protein function prediction method is still a significant challenge.
- Deep learning is a powerful tool that, with sufficient amounts of data, can take proteomics far further than current methods.
To be continued…
The next part in this series will summarize the recent adoption of deep learning advancements in proteomics, which is slowly leading to a better understanding of (microbial) proteins.Bibliography
[1] N. Koppel and E. P. Balskus, “Exploring and Understanding the Biochemical Diversity of the Human Microbiota,” Cell Chem Biol, vol. 23, no. 1, pp. 18–30, Jan. 2016, doi: 10.1016/j.chembiol.2015.12.008.
[2] P. Amon and I. Sanderson, “What is the microbiome?,” Archives of Disease in Childhood – Education and Practice, vol. 102, no. 5, pp. 257–260, Oct. 2017, doi: 10.1136/archdischild-2016-311643.
[3] “CAFA | Bio Function Prediction.” [Online]. Available: https://www.biofunctionprediction.org/cafa/. [Accessed: 30-Apr-2020].
[4] “Home – Prediction Center.” [Online]. Available: http://predictioncenter.org/. [Accessed: 30-Apr-2020].
[5] S. F. Altschul, W. Gish, W. Miller, E. W. Myers, and D. J. Lipman, “Basic local alignment search tool,” J. Mol. Biol., vol. 215, no. 3, pp. 403–410, Oct. 1990, doi: 10.1016/S0022-2836(05)80360-2.
[6] S. F. Altschul et al., “Gapped BLAST and PSI-BLAST: a new generation of protein database search programs,” Nucleic Acids Res., vol. 25, no. 17, pp. 3389–3402, Sep. 1997, doi: 10.1093/nar/25.17.3389.
[7] S. R. Eddy, “Profile hidden Markov models,” Bioinformatics, vol. 14, no. 9, pp. 755–763, 1998, doi: 10.1093/bioinformatics/14.9.755.
[8] M. Steinegger, M. Meier, M. Mirdita, H. Vöhringer, S. J. Haunsberger, and J. Söding, “HH-suite3 for fast remote homology detection and deep protein annotation,” BMC Bioinformatics, vol. 20, no. 1. 2019, doi: 10.1186/s12859-019-3019-7.
[9] Z. D. Ariel Schwartz, “Deep Learning Applied to Genomics, Deep Semantic Protein Representation.”
[10] C. Angermueller, T. Pärnamaa, L. Parts, and O. Stegle, “Deep learning for computational biology,” Mol. Syst. Biol., vol. 12, no. 7, p. 878, Jul. 2016, doi: 10.15252/msb.20156651.
[11] A. W. Senior et al., “Improved protein structure prediction using potentials from deep learning,” Nature, vol. 577, no. 7792, pp. 706–710, Jan. 2020, doi: 10.1038/s41586-019-1923-7.
[12] A. W. Senior et al., “Protein structure prediction using multiple deep neural networks in the 13th Critical Assessment of Protein Structure Prediction (CASP13),” Proteins, vol. 87, no. 12, pp. 1141–1148, Dec. 2019, doi: 10.1002/prot.25834.
[13] A. Kryshtafovych, T. Schwede, M. Topf, K. Fidelis, and J. Moult, “Critical assessment of methods of protein structure prediction (CASP)-Round XIII,” Proteins, vol. 87, no. 12, pp. 1011–1020, Dec. 2019, doi: 10.1002/prot.25823.
[14] T. Ching et al., “Opportunities and obstacles for deep learning in biology and medicine: 2019 update.” [Online]. Available: https://greenelab.github.io/deep-review/. [Accessed: 28-Dec-2018].
[15] V. Boža, B. Brejová, and T. Vinař, “DeepNano: Deep recurrent neural networks for base calling in MinION nanopore reads,” PLoS One, vol. 12, no. 6, p. e0178751, Jun. 2017, doi: 10.1371/journal.pone.0178751.
[16] A. Bateman et al., “UniProt: the universal protein knowledgebase,” Nucleic Acids Res., vol. 45, no. D1, pp. D158–D169, Jan. 2017, doi: 10.1093/nar/gkw1099.