In this blog post, we discuss artificial intelligence (AI) and machine learning (ML) methods for High Content Screening (HSC) data analysis and highlight how these methods enhance data processing, quality control and feature extraction to support drug discovery and development.
High Content Screening (HCS) in Drug Discovery
High Content Screening (HSC) is an imaging and quantification method that enables simultaneous evaluation of multiple cellular and molecular features and supports unbiased phenotypic screening, making it a valuable tool for drug discovery and development. The advances in cell imaging and computational image analysis methods have propelled the field forward over the last decade and enabled research and clinical insights. Today, artificial intelligence (AI) and machine learning (ML) are further expanding the capabilities of this approach to accelerate drug discovery and development.
One of the main advantages of HCS is the ability to test potential drug candidates directly in living systems that mimic disease states. Such information-rich data facilitates comprehensive assessment of the effect of active molecules on cells and produces insights relevant to clinical outcomes. HSC can be used across many steps of the drug discovery pipeline, including early stages of discovery, lead optimization and target validation, as well as in toxicity assessment, drug repurposing and basic research. HCS enables researchers to screen thousands of active compounds in a single experiment. It can help identify potential targets of therapeutic intervention, as well as elucidate the modes and mechanisms of action of drug compounds. In toxicity screening, HCS can be used to detect adverse effects on cell viability and function.
While HCS is a powerful approach, the method is not without challenges and limitations. The sheer size and complexity of HCS data presents a big hurdle for deconvoluting and extracting insights from screening datasets. Custom assays take a long time to develop, and the output requires sophisticated data analysis methods that are difficult to automate and generalize. Variation in imaging methods, as well as biological variability and complexity also contribute to the challenges of HCS application. ML approaches solve many of these challenges and unlock the insights in HCS data by enhancing quality control (QC), facilitating feature extraction and introducing multimodal capabilities for better predictions.
What Is Cell Painting?
One of the most common HCS assays is Cell Painting [1]. Cell Painting is a morphological profiling assay that uses six fluorescent dyes to label eight different components of the cell like nucleus, endoplasmic reticulum, Golgi, mitochondria, lysosomes, endosomes, as well as the cytoskeleton. It is capable of capturing thousands of metrics and features in imaged cells. Analyzing these images enables visual detection of the spatial relationship between organelles or morphological changes that occur in response to drug treatment.
Compared to specialized, conventional HCS assays which can take months or even years to develop, Cell Painting provides a ready-to-use solution for comprehensive and unbiased data capture and can be scaled to test up to hundreds of thousands of compounds. Cell Painting inexpensively combines multiple stains in a robust assay to reveal thousands of morphological features. Because Cell Painting is a standardized method used by many researchers, the data collected can be easily shared, combined and repurposed by different teams for various applications.
Thanks to its quick deployment, high throughput and richness of readout, Cell Painting is widely used in initial drug discovery screening studies, providing unbiased and diverse information about the effects of screened compounds on cells. Cell Painting has been used for elucidating the mechanism of action (MOA) [2] and bioactivity [3]. It is an attractive solution for toxicology, reducing the use of animals in testing [4]. Additionally, Cell Painting has been used in structure-activity relationships (SAR) studies to assess the biological activity of newly synthesized compounds and to build diversity sets for focused libraries [5].
JUMP-Cell Painting (JUMP-CP) Consortium, an organization supported by the Massachusetts Life Sciences Center (MLSC), has put together a large public data set to encourage the development of phenotypic drug discovery approaches. Ardigen is a JUMP-CP supporting partner and has contributed to supporting the goals of the consortium by developing the JUMP-CP Data Explorer tool.
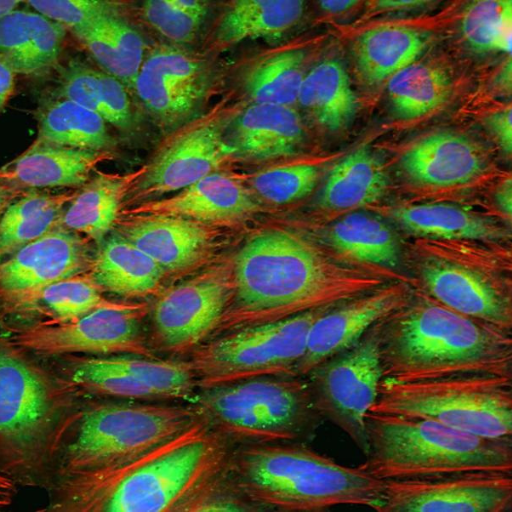
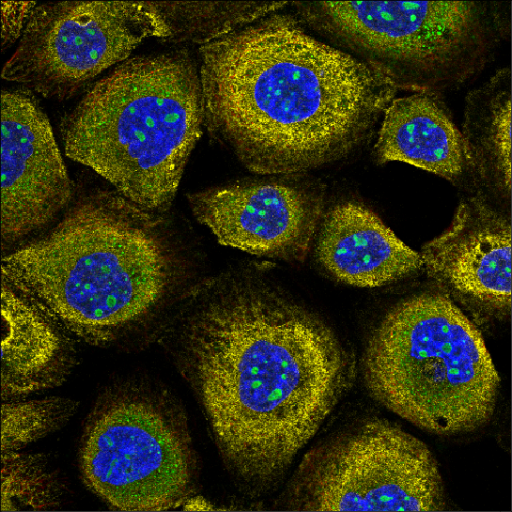
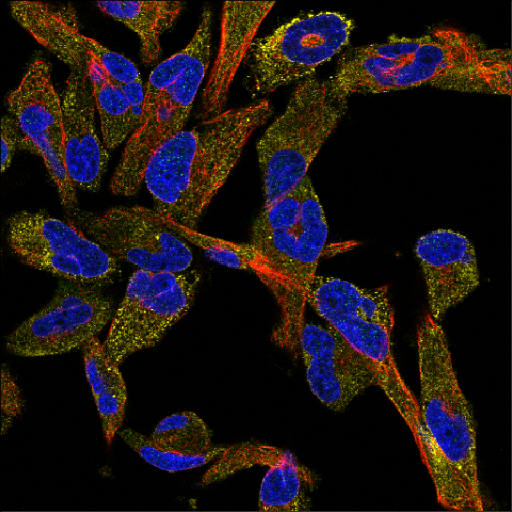
How Can AI Aid in High Content Screening Data Analysis?
Data analysis has historically been a challenge for HCS application. The biological complexity, high-dimensionality and other reasons mentioned above have restricted the insights that can be gained from HCS datasets. However, advances in ML and AI are powering a resurgence of interest in image-based profiling. Automated image analysis methods, such as computer vision, are developing rapidly [6]. Complex functions such as segmentation and feature extraction are also increasingly shifting from human-defined to deep learning-based [7]. A detailed overview of recent advances in these approaches is presented in this review [8].
Increasingly, ML approaches have contributed to expanding the range of applications of HCS assays, such as repurposing existing datasets for predicting the activity of compounds in other assay scenarios. For instance, in a multi-institution study an HCS dataset from Janssen was used to successfully predict the activity of structurally diverse compounds, increasing hit rates by 60- to 250-fold compared with the original screening assays [9]. This study used CellProfiler software for image segmentation and feature extraction, followed by supervised machine learning for activity prediction. A follow-up study used the developed framework to annotate a Cell Painting dataset of 30,000 compounds [10].
Experts estimate that deep learning may therefore eventually replace classical image processing and feature extraction methods [11]. ML image processing is capable of recognizing and extracting image characteristics that go beyond human-defined features. Additionally, the extracted features are aggregated into profiles using unbiased methods according to biologically meaningful similarities.
ML analysis results are heavily influenced by the computational frameworks chosen to perform the task. Neural networks, which are machine-learning methods defined by flexible architecture that use weighted features to learn to distinguish features, are among the most widely used approaches. Deep convolutional neural networks can integrate bespoke feature extraction and interpretive tasks in a single process [12] as well as capture single-cell heterogeneity [13].
Using AI for Phenotypic Drug Discovery
AI methods can be used to automate image analysis, feature extraction and sample clustering. This leads to a significant reduction in the time and cost of data analysis, increased throughput and improved, unbiased decision making. Many pharma companies are leveraging existing datasets to extract novel insights through ML, rekindling industry interest in phenotypic profiling for drug discovery.
Developing high-quality ML analysis pipelines requires significant expertise in HCS methods, as well as machine learning, software and data science. The algorithms are constantly evolving and require specialized knowledge, which is why we have developed a dedicated platform for analyzing HCS data. Ardigen phenAID has helped companies to improve their analysis time and enhance the quality of predictions by combining multiple data modalities, including images and chemical structure.
If you are curious to learn more about Ardigen phenAID platform, visit our website. For insights into how Ardigen phenAID analysis boosted the quality of predictions on a Merck phenotyping dataset, download our poster.
Works Cited:
- Bray, M.-A. et al. (2016c). “Cell Painting, a High-content Image-based Assay for Morphological Profiling Using Multiplexed Fluorescent Dyes,” Nature Protocols. Available at: https://pubmed.ncbi.nlm.nih.gov/27560178/
- Cox, M.J. et al. (2020). “Tales of 1,008 small molecules: phenomic profiling through live-cell imaging in a panel of reporter cell lines,” Scientific Reports. Available at: https://pubmed.ncbi.nlm.nih.gov/32764586/
- Nyffeler, J. et al. (2020). “Bioactivity screening of environmental chemicals using imaging-based high-throughput phenotypic profiling,” Toxicology and Applied Pharmacology. Available at: https://www.sciencedirect.com/science/article/abs/pii/S0041008X19304843
- Thomas, R.S. et al. (2019). “The next generation blueprint of Computational Toxicology at the U.S. Environmental Protection Agency,” Toxicological Sciences. Available at: https://academic.oup.com/toxsci/article/169/2/317/5369737
- Gerry, C.J. et al. (2016). “Real-Time biological annotation of synthetic compounds,” Journal of the American Chemical Society. Available at: https://pubs.acs.org/doi/full/10.1021/jacs.6b04614
- Grys, Ben T., et al. (2017). “Machine learning and computer vision approaches for phenotypic profiling,” Journal of Cell Biology. Available at: https://rupress.org/jcb/article/216/1/65/46129/Machine-learning-and-computer-vision-approaches
- Caicedo, J.C. et al. (2017). “Data-analysis strategies for image-based cell profiling,” Nature Methods. Available at: https://www.nature.com/articles/nmeth.4397
- Chandrasekaran, S.N. et al. (2020). “Image-based profiling for drug discovery: due for a machine-learning upgrade?” Nature Reviews Drug Discovery. Available at: https://www.nature.com/articles/s41573-020-00117-w
- Simm, J., et al. (2018). “Repurposing high-throughput image assays enables biological activity prediction for drug discovery,” Cell Chemical Biology. Available at: https://www.cell.com/cell-chemical-biology/fulltext/S2451-9456(18)30037-0
- Bray, M.-A. et al. (2017). “A dataset of images and morphological profiles of 30 000 small-molecule treatments using the Cell Painting assay,” Gigascience. Available at: https://academic.oup.com/gigascience/article/6/12/giw014/2865213
- McQuin, C. et al. (2018). “CellProfiler 3.0: Next-generation image processing for biology,” PLoS Biology. Available at: https://journals.plos.org/plosbiology/article?id=10.1371/journal.pbio.2005970
- Kensert, A., et al. (2019). “Transfer Learning with Deep Convolutional Neural Networks for Classifying Cellular Morphological Changes,” SLAS Discovery. Available at: https://journals.sagepub.com/doi/full/10.1177/2472555218818756
- Rohban, M.H. et al. (2019). “Capturing single-cell heterogeneity via data fusion improves image-based profiling,” Nature Communications. Available at: https://www.nature.com/articles/s41467-019-10154-8