Exploring multimodal AI for personalized medicine
In the pursuit of precision medicine, identifying treatment responders remains a challenge. By integrating multiple data sources—histology, clinical information, and AI—we can enhance predictive capabilities and drive better patient outcomes.
Challenge
The client needed a reliable approach to differentiate responders from non-responders using clinical and histology data. Ensuring fair validation and optimal data utilization was a key requirement.
Approach
- Explored multimodal AI fusion techniques.
- Designed a rigorous validation process with extensive patient stratification.
- Combined tissue morphology graphs, histology images, and clinical data.
- Applied various deep learning methodologies for accurate predictions.
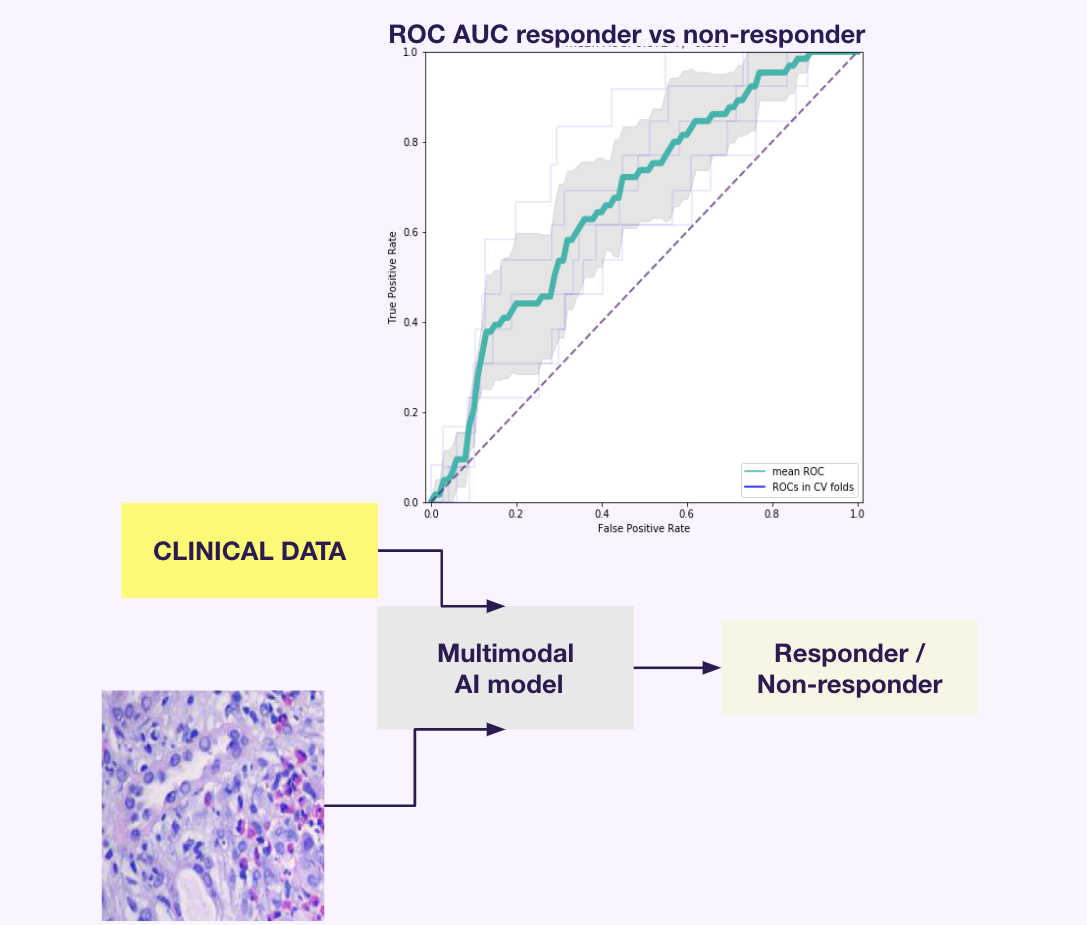
Results
- Achieved over a 10% improvement in ROC AUC compared to the baseline model.
- Identified the most predictive data modalities for response differentiation.
- Ensured high reliability through a strict validation schema.
This AI-driven approach enables more effective patient stratification, improving treatment outcomes through precise responder identification.